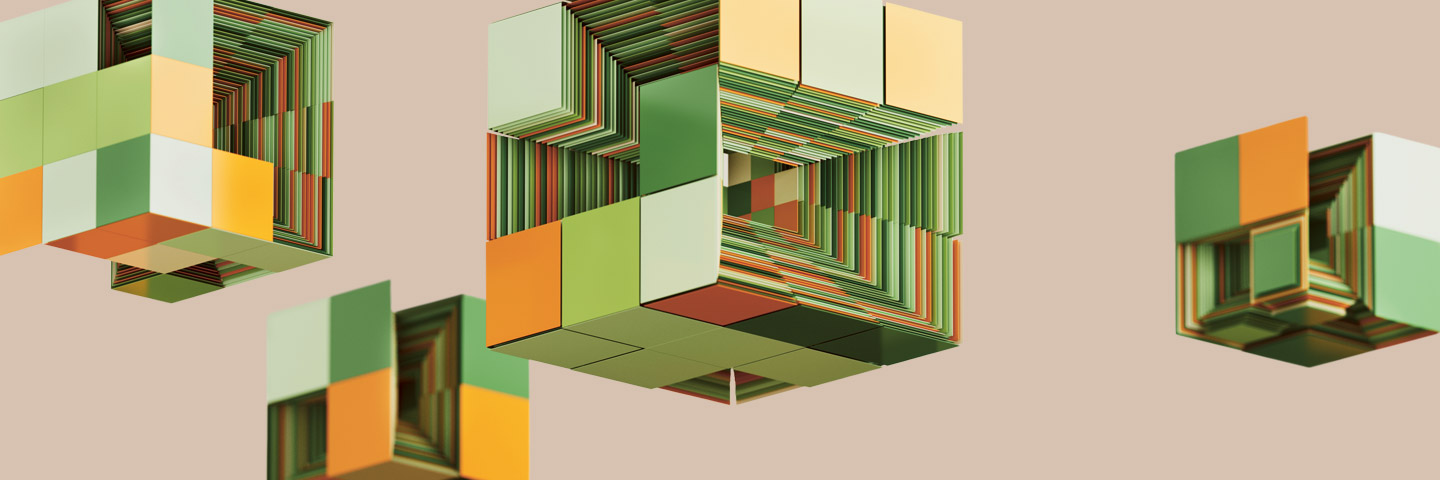
Synthetic data in research.
One of the driving forces for the use of synthetic data is the company DataCebo that emerged from a research project at the Massachusetts Institute of Technology. When a research team in 2012 wanted to study the behaviour of 155,000 participants in online courses in an attempt to find out why students weren’t finishing their courses, the data was not accessible due to data protection regulations. In order to still achieve practical results, the researchers created synthetic course participants that could no longer be identified but behaved exactly the same as the real participants. This way the team could carry on with their research while adhering to data protection regulations.
In the name of equality.
Synthetic data can also play a role in combating discrimination. It is known that AI modules reproduce biases and inequalities if these are anchored in their training data, for example image recognition AI that is primarily trained with Caucasian faces and hence discriminates against people of colour. Synthetic data can make a difference, as it contains a large amount of artificially generated faces that make the dataset more diverse and representative.
Jesper Kleinjohann and tech journalist Svea Eckert talk about the future of optical character recognition, mixing natural and synthetic data and the ambiguity of texts.
Training language models.
Synthetic data is needed to feed data-hungry large AI developers such as OpenAI. We have seen the development of language models that have been trained with synthetic ChatGPT conversations, which imitate the answers of the AI and are hence an imitation of an imitation of human conversations. The results, at first, are astoundingly realistic, but upon closer inspection show a tendency to reproducing false information, making it essential to check and validate the use of synthetic data.
Healthcare
In medicine, access to a sufficient amount of high-quality data is a major problem, even more so when it comes to rare illnesses or unusual phenomena. Here, previously generated synthetic data can help to provide an adequate dataset for diagnoses, predictions and possible treatment methods.
Decisive for corporations.
Even in business and industry, synthetic data can be put to a large number of uses. Companies make decisions based on data to optimise their business processes, which are often sparsely documented. By means of process mining, these empty spaces are filled up with computer models and the processes are documented very realistically. Model calculation has been a common practice in finance for decades, by using synthetic data for risk evaluation, portfolio management and predicting market developments. In business, this means whoever has the best data has the competitive edge.
As early as 2024, 60 per cent of all data used for AI and analytics projects will be synthetic.
Source: Gartner
Turbo-charging autonomous driving.
Autonomous driving is only developing slowly, at least on the surface. Did you know that synthetic data can be used as a means to boost this development? It allows the creation of virtual traffic scenarios in which AI models can be trained under realistic conditions.
More affordable.
Collecting real data is often difficult and expensive. The risk of violating intellectual property rights or data protection regulations can result in additional costs. Producing it synthetically is less risky and the algorithms save money hence, AI models are more economically feasible, allowing small companies and research facilities to keep up with the top dogs.
A new era?
Despite the many advantages, synthetic data should never replace real data, but rather complement or supplement an existing source. For it to be used effectively, synthetic data must be as realistic and representative as possible for the use case, which makes developing and generating this data increasingly difficult. Once achieved, however, the combination of synthetic and real data allows more significant analyses and better AI models.

This article was printed in Bechtle update 02/2023. Find out more on page 38.